Advantages of Machine Learning In Healthcare Industry
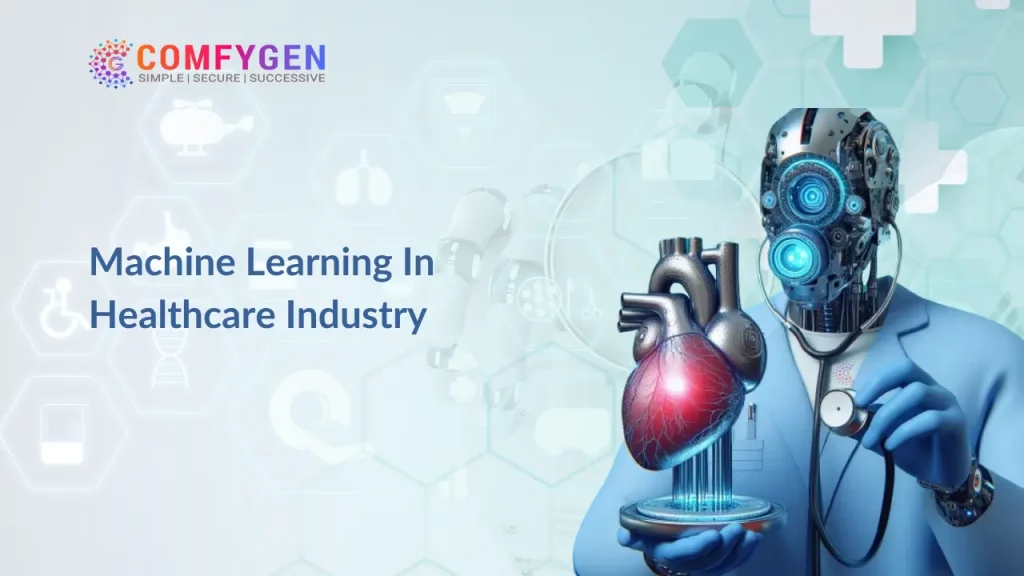
Healthcare industry has brought significant change in its operations and boosted its efficiency impeccably with the implementations with several advanced technologies implementations. One of the most impactful advanced technologies was machine learning in healthcare, which challenged all the loopholes left and brought revolutionary changes into that.
With the help of machine learning (aka. ML); the researchers could make the drug discoveries easier, patients can get better outcomes from treatments, healthcare professionals can treat patients with more accuracy, the data collection and storage have become much more convenient, et cetera. ML helped the industry in several ways, and still the industry hoped to see some more evolutions with the help of this dynamic technology.
This blog is going to explain whole scenarios around ML in the Healthcare Industry. We will tell you about its impact, its evolutions, the top-notch running applications, benefits, and more. Let’s stick to the blog, and gain knowledge that is good for everyone.
What Is Machine Learning In Healthcare?
Machine learning in healthcare refers to the application of artificial intelligence (AI) algorithms that allow computers to learn from and make predictions based on medical data. These algorithms analyze vast amounts of patient information, including electronic health records (EHRs) machine learning, medical images, and genetic data, to uncover patterns and insights that can assist healthcare professionals in diagnosing diseases, predicting patient outcomes, and personalizing treatment plans.
The integration of machine learning in healthcare is revolutionizing the industry by improving accuracy, efficiency, and patient care. For example, predictive models can identify patients at risk of developing certain conditions, enabling early intervention and prevention. Additionally, machine learning aids in the development of new treatments by analyzing clinical trial data and accelerating the drug discovery process. By harnessing the power of machine learning, healthcare providers can make more informed decisions, ultimately leading to better health outcomes and a more efficient healthcare system.
Use Cases Of Machine Learning In Healthcare
The machine learning development services have helped the healthcare industry in numerous ways. Even more, it brought many use-cases into the industry. Some are listed right below, read them:
- Patient Behavior Modification
Machine learning algorithms can analyze patient data to identify unhealthy behaviors and predict potential health risks. By providing personalized feedback and recommendations, healthcare providers can encourage patients to adopt healthier lifestyles. For example, wearable devices can monitor physical activity and vital signs, prompting users to exercise more or adhere to medication schedules, ultimately leading to better health outcomes.
- Virtual Nursing
Virtual nursing assistants, powered by healthcare machine learning, can interact with patients via chatbots or voice assistants to answer questions, provide medication reminders, and offer medical advice. These AI-driven tools can reduce the burden on healthcare professionals, improve patient engagement, and ensure continuous monitoring of patients, especially those with chronic conditions, enhancing overall patient care and support.
- Disease Outbreak Predictions
Machine learning models can analyze data from various sources, including social media, travel patterns, and public health reports, to predict and track disease outbreaks. By identifying trends and anomalies in real-time, these models enable public health officials to respond more quickly to emerging threats, implement preventive measures, and allocate resources effectively to mitigate the spread of infectious diseases.
- Medical Imaging
Machine learning algorithms can assist radiologists by analyzing medical images, such as X-rays, MRIs, and CT scans, to detect abnormalities and diagnose conditions with high accuracy. These tools can identify early signs of diseases like cancer, reducing diagnostic errors and enabling timely treatment. Additionally, they can streamline the workflow, allowing radiologists to focus on complex cases that require human expertise.
- Hospital Management Optimization
Machine learning can optimize hospital operations by predicting patient admission rates, optimizing staff schedules, and managing inventory. By analyzing historical data and current trends, hospitals can improve resource allocation, reduce wait times, and enhance patient flow. This leads to more efficient operations, cost savings, and improved patient experiences, ultimately contributing to better healthcare delivery.
- Accurate Diseases
Machine learning healthcare models can improve the accuracy of disease diagnoses by analyzing patient data ML, including symptoms, medical history, and test results. These models can recognize patterns and correlations that might be missed by human practitioners, leading to more accurate and timely diagnoses. This enhances patient outcomes by ensuring appropriate and effective treatments are administered promptly.
- Identifying High-Risk in Patient’s Conditions
Machine learning can identify patients at high risk of developing severe conditions by analyzing a wide range of data points, such as genetic information, lifestyle factors, and clinical records machine learning. Predictive models can alert healthcare providers to potential complications, allowing for early intervention and personalized care plans. This proactive approach can prevent the progression of diseases and improve patient prognosis.
- Robot-Assisted Surgeries
Machine learning enhances robot-assisted surgeries by providing real-time data and precision guidance to surgeons. These advanced systems can analyze patient-specific data to plan and execute surgical procedures with high accuracy, reducing the risk of complications and improving recovery times. By augmenting the skills of surgeons, machine learning helps achieve better surgical outcomes and patient safety.
- Drug Discovery
Machine learning accelerates drug discovery by analyzing vast datasets to identify potential drug candidates and predict their efficacy and safety. By modeling complex biological processes, these algorithms can uncover new therapeutic targets and optimize the drug development process. This reduces the time and cost associated with bringing new medications to market, ultimately leading to innovative treatments for various diseases.
Want To Integrate Machine Learning Into Your Healthcare App
The Future of Doctors: Will Machine Learning Replace Them?
As artificial intelligence and robotics continue to improve, the idea that robots will eventually replace doctors is becoming more popular. But it’s doubtful that technology will ever completely replace physicians. Healthcare cannot function without the human touch, even if AI may help with illness diagnosis, image analysis, and even some forms of surgery. Machines cannot replace human traits like empathy, intuition, and the capacity to comprehend complex patient problems. To ensure appropriate therapy, clinicians also bring a thorough awareness of the patient’s personal background and medical history.
Without a question, machines may improve healthcare by simplifying routine chores and offering precise data analysis, freeing up clinicians to concentrate more on patient care. But the future of medical care lies on the combination of human physicians and cutting-edge technology. Healthcare is made more effective and efficient by this synergy, which allows clinicians to give patients the individualized treatment and vital human connection that they require, while machines tackle data-driven duties.
Benefits Of Machine Learning In Healthcare
Machine learning benefitted the healthcare industry in several ways. Here are some of the benefits showcased to let readers understand how ML is efficient for the healthcare industry:
- Enhanced Patient Experiences
Machine learning enhances patient experiences by providing personalized care and improving communication. AI-driven tools can offer tailored health recommendations, doctor appointment reminders, and follow-up care instructions, ensuring patients feel supported and informed throughout their healthcare journey. Additionally, machine learning can streamline administrative processes, reducing wait times and enhancing overall patient satisfaction by making healthcare interactions more efficient and responsive.
- Automated Routine Tasks
Machine learning automates routine tasks such as data entry, appointment scheduling, and billing, freeing healthcare professionals to focus on patient care. By reducing the administrative burden, healthcare providers can spend more time on clinical duties and direct patient interactions. Automation also minimizes human errors in repetitive tasks, ensuring more accurate and efficient operations within healthcare facilities.
- Reduced Risks
Machine learning reduces risks in healthcare by improving diagnostic accuracy and predicting potential complications. Predictive analytics can identify patients at high risk for conditions like heart disease or diabetes, enabling early intervention. Additionally, AI-driven tools can detect errors in medication prescriptions and prevent adverse drug interactions, enhancing patient safety and minimizing the likelihood of medical mistakes.
- Improved Decision-Making Power
Machine learning enhances decision-making by providing healthcare professionals with data-driven insights and recommendations. By analyzing vast amounts of patient data, machine learning models can identify patterns and trends that inform clinical decisions. This leads to more accurate diagnoses, effective treatment plans, and better patient outcomes. Machine learning Healthcare providers can rely on these advanced tools to make informed, evidence-based decisions quickly and confidently.
- Accelerate the Innovation Power
Machine learning accelerates innovation in healthcare by facilitating research and development. AI algorithms can analyze large datasets to uncover new insights into diseases, treatment methods, and patient responses. This accelerates the discovery of novel therapies, medical devices, and diagnostic tools. Machine learning also enables rapid testing and iteration, shortening the time from research to clinical application of machine learning and fostering continuous improvement in healthcare technologies.
- Extended Healthcare Accessibility
Machine learning extends healthcare accessibility by enabling remote monitoring and telemedicine service. AI-powered platforms can provide medical consultations, monitor chronic conditions, and offer health advice to patients in underserved or remote areas. This reduces barriers to healthcare access, ensuring that more people receive timely and appropriate care. Additionally, machine learning can optimize resource allocation in healthcare systems, making services more available and affordable to a broader population.
Related Blog: Top Trends In Healthcare App Development For 2024
Difference Between Machine Learning And Deep Learning in Healthcare Organization
Feature | Machine Learning (ML) | Deep Learning (DL) |
---|---|---|
Definition | A subset of AI that uses algorithms to learn from data and make predictions. | A subset of ML that uses neural networks with many layers to learn from large amounts of data. |
Data Requirements | Effective with smaller datasets; performance improves with labeled data. | Requires large datasets; performs better with extensive, labeled data. |
Complexity | Simpler algorithms (e.g., decision trees, SVMs) with fewer parameters. | Highly complex with multiple layers and millions of parameters. |
Feature Engineering | Requires manual feature extraction and selection by experts. | Automatically extracts and learns features from raw data. |
Processing Power | Lower computational requirements; can run on standard CPUs. | High computational requirements; typically require GPUs for training. |
Applications in Healthcare | Disease prediction, patient outcome prediction, and treatment recommendation systems. | Medical image analysis (e.g., MRI, CT scans), drug discovery and genomics. |
Interpretability | Generally more interpretable; easier to understand and explain decisions. | Often seen as a “black box”; harder to interpret and explain decisions. |
Training Time | Generally faster training times. | Requires longer training times due to complex architectures. |
Accuracy | Good accuracy with well-prepared data. | Higher accuracy, especially with large, diverse datasets. |
Use Cases | Predicting hospital readmissions, identifying at-risk patients, and optimizing resource allocation. | Detecting tumors in medical imaging, personalized medicine, and analyzing genetic data. |
Top-Notch Healthcare Apps Using ML
Here are the top-notch machine learning apps in the healthcare industry. Know these and its efficiency:
- Viz.ai
Viz.ai uses advanced technology to improve how healthcare teams collaborate. It helps frontline healthcare providers quickly connect with specialists, which speeds up decision-making and leads to better outcomes for patients.
- Deep Genomics
Deep Genomics uses artificial intelligence to speed up drug discovery for genetic diseases. Their platform analyzes genetic data to understand how mutations cause diseases. It can also quickly assess millions of potential medicines, helping researchers find the most effective treatments faster.
- Intuitive Surgical
Intuitive Surgical developed the Da Vinci Surgical System, which uses machine learning to assist surgeons in performing minimally invasive surgeries. This technology enhances surgical precision and improves patient recovery times.
- Oncora Medical
Oncora Medical focuses on improving cancer treatment and research. They gather data from various sources like medical records and cancer registries to evaluate treatment effectiveness. Their approach to developing machine learning models mirrors that of custom pharmaceutical software companies, ensuring rigorous testing and validation.
- PathAI
PathAI supports pathologists by using machine learning to aid in diagnosing diseases from patient tissue samples. They develop algorithms that can analyze large amounts of data, helping pathologists make more accurate diagnostic decisions and improving patient care.
Upgrade Your Healthcare App Today
Challenges Of Adapting Machine Learning In Healthcare
Implementing machine learning in Healthcare also has some challenges along with benefits and numerous efficient use-cases; such as:
- Biased Datasets
Machine learning models can inherit biases present in the datasets they are trained on. If the data lacks diversity or reflects historical biases, the AI system may produce skewed results, potentially leading to disparities in healthcare outcomes. Addressing this issue requires careful dataset curation, diverse representation, and ongoing monitoring to ensure fair, unbiased AI, and machine learning applications in healthcare.
- Patient Safety
Ensuring patient safety is a critical challenge when implementing artificial intelligence and machine learning in health. AI models must be rigorously validated to prevent errors in diagnosis or treatment recommendations. Even minor inaccuracies can have significant consequences for patient health. Continuous evaluation, robust testing, and regulatory oversight are necessary to maintain high standards of safety and reliability in AI-driven healthcare solutions.
- Privacy Concerns
Machine learning development in healthcare often involves processing vast amounts of sensitive patient data. Ensuring the privacy and security of this information is paramount. Breaches or misuse of data can lead to severe consequences, including loss of patient trust and legal repercussions. Implementing stringent data protection measures, adhering to regulations like HIPAA, and using anonymization techniques can help mitigate privacy concerns.
- Integration with Existing Systems
Integrating machine learning solutions with existing healthcare systems can be challenging. Many healthcare providers use legacy systems that may not be compatible with modern AI technologies. This integration requires significant investment in infrastructure, training, and change management. Ensuring seamless interoperability while maintaining the continuity of care is crucial for successful adoption.
- Regulatory Hurdles
The regulatory landscape for machine learning in healthcare is complex and still evolving. AI applications must meet strict regulatory standards to ensure safety and efficacy. Navigating these regulations can be time-consuming and costly. Healthcare app developers must work closely with regulatory bodies to achieve compliance and ensure that their solutions are safe for clinical use.
- Ethical Considerations
The use of AI in healthcare raises ethical concerns, such as decision-making transparency and accountability. Patients and healthcare providers need to trust that AI recommendations are based on sound reasoning and ethical principles. Ensuring transparency in AI algorithms and maintaining human oversight in critical decision-making processes are essential to address these ethical challenges.
Also Read: The Comprehensive Guide to Healthcare App Development
Future Of Machine Learning In Healthcare
The future of machine learning (ML) in healthcare is poised to revolutionize the industry, making medical care more personalized, efficient, and proactive. As technology advances, ML will increasingly enable healthcare providers to deliver highly individualized treatment plans by analyzing vast amounts of patient data, including genetic information, lifestyle factors, and historical health records. This will lead to more accurate diagnoses and tailored therapies, improving patient outcomes significantly.
One of the most exciting prospects is the integration of ML with wearable technology and remote monitoring systems. These devices will continuously collect health data, allowing ML algorithms to detect early signs of potential health issues and alert both patients and healthcare providers. This proactive approach can prevent the escalation of chronic conditions and facilitate timely interventions, ultimately enhancing the quality of life for many individuals.
Additionally, ML will play a crucial role in advancing precision medicine. By analyzing the genetic makeup of patients, ML can identify specific mutations and suggest targeted treatments that are more likely to be effective. This will revolutionize fields such as oncology, where personalized cancer treatments can be developed based on the unique genetic profile of each patient’s tumor.
Moreover, ML-driven automation will streamline administrative processes and reduce the burden on healthcare professionals. Tasks such as medical coding, billing, and appointment scheduling can be automated, freeing up valuable time for doctors and nurses to focus on patient care.
However, the widespread adoption of ML in healthcare will require addressing challenges such as data privacy, ethical considerations, and the need for robust regulatory frameworks. As these issues are resolved, the future of ML in healthcare promises a more efficient, accurate, and patient-centric approach to medical care, ultimately transforming the healthcare landscape for the better.
Are You Looking For A Company That Specializes In Healthcare Machine Learning?
Contact Us:
WhatsApp: +91 958-786-7258
Email: [email protected]
Telegram: @comfygen
Final Verdict
Machine learning is set to revolutionize healthcare, offering unprecedented opportunities for personalized care, improved diagnostics, and operational efficiency. By harnessing the power of AI, healthcare providers can deliver more accurate and timely treatments, enhance patient outcomes, and streamline administrative processes.
Despite the challenges such as data privacy, biased datasets, and regulatory hurdles, the potential benefits far outweigh the drawbacks. As technology advances and ethical considerations are addressed, the integration of machine learning into healthcare systems will become more seamless and widespread. The future promises a more proactive, patient-centric approach to medical care, where technology and human expertise work hand-in-hand to deliver optimal health outcomes.
Embracing machine learning in healthcare is not just an innovation—it’s a necessary evolution towards a smarter, more efficient, and compassionate healthcare system.
Frequently Asked Questions (FAQs)
How much does it cost to integrate ML in healthcare?
How can I hire the best machine learning development company for healthcare industry?
What should I consider when implementing machine learning in healthcare?
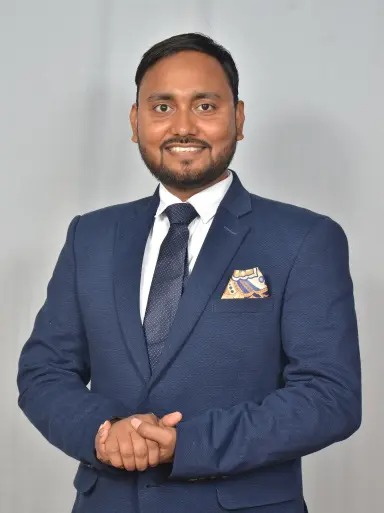
Mr. Saddam Husen, (CTO)
Mr. Saddam Husen, CTO at Comfygen, is a renowned Blockchain expert and IT consultant with extensive experience in blockchain development, crypto wallets, DeFi, ICOs, and smart contracts. Passionate about digital transformation, he helps businesses harness blockchain technology’s potential, driving innovation and enhancing IT infrastructure for global success.
Based on Interest
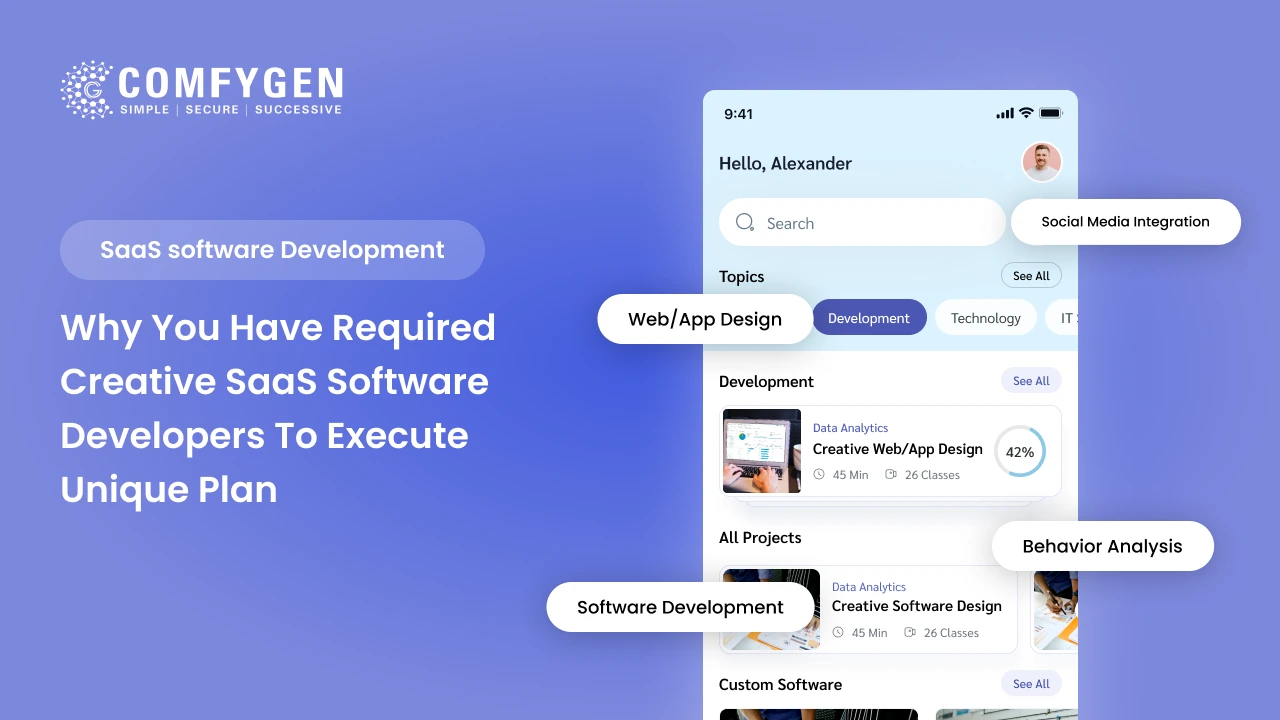
Why You Need Creative SaaS Software Developers for a Unique Project?
Businesses across industries are adapting Software-as-a-Service (SaaS) software solutions to streamline organization operations, improve customer experiences, and gain a competitive edge. However,…